
Empowering SMEs: How Technology is Reshaping the Digital Lending Space

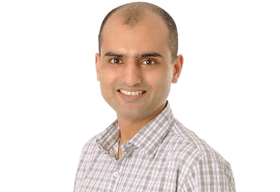
The last decade has seen rapid technological advancements, mostly driven by the internet and smartphones. The influx of sophisticated technologies such as artificial intelligence, machine learning, IoT and Big Data is further drivingthe digital journey. This in turn has resulted in a proliferation of data. According to industry estimates, 2.5 quintillion bytes of data are created every day and the pace is accelerating. International Data Corporation's (IDC) projections suggest that the global datasphere will grow from 33 zettabytes in 2018 to 175 zettabytes by 2025 (1 zettabyte is equivalent to a trillion gigabytes). The data explosion has benefitted segments of the population and several industries that previously didn’t have access to formal credit, thereby boosting financial inclusion across India.
Role of ML & Predictive Analysis in Fraud Detection
The lending space in India has seen a spike in loan frauds. Data released by the RBI reveals that loan frauds increased from Rs. 22,558 crore in fiscal 2018 to Rs. 64,548 crore in 2019. Machine learning and predictive analysis can prove to be particularly effective in identifying fraudulent patterns in existing datasets and then applying the insights to spot future fraud attempts. The advantage machine learning has over other technologies available for identifying fraud activities is that it has the ability to learn continually. The more data a machine learning system is fed, the more accurate its predictions will be. By analyzing vast amounts of both traditional and alternative data, it can then learn the difference between
legitimate and fraudulent transactions based on a contextual intelligence perspective.
AI-based Credit Underwriting for Faster Loan Processing
Modern technologies can automate and streamline the credit underwriting process. Financial institutes have traditionally been using a manual process for scoring and approving or rejecting loans, wherein analysts score each loan against a set of financial metrics that are in place to determine the acceptability of the borrower. The manual process of credit scoring is time-consuming and often leads to mistakes and biased decisions. In contrast, an AI-powered credit underwriting model combines traditional data with alternative data, which includes the borrower’s digital footprint, daily transactions, payments history and even legal case history, to provide accurate credit assessment in near realtime. Besides facilitating faster processing and approval of loans, AI-based scoring systems enable credit underwriters to focus on more complex issues that might requirehuman intervention.
Integration of Machine Learning for Accurate Credit Risk Analysis
Credit-risk analysis allows financial institutes to assess the possibility of the borrower’s failure to repay the loans and the amount of loss caused by the same. If done manually, credit-risk analysis can not only take up to days, but also is more prone to mistakes. This is where machine learning has a crucial role to play. ML-based credit analysis models can identify complex, nonlinear patterns within large sets of data, thereby enabling more accurate credit-risk analysis.Such analysis can help lenders to understand the true credit-worthiness of the borrower and reduce default cases to a great extent. Some of the powerful algorithms that banks, Fintechs and NFBCs can use to weed out bad cases early are artificial neural networks, random forest and boosting.
How does this benefit the SMEs?
Lending to small and medium enterprises poses a major challenge for lenders in India. That’s because lenders have little or no access to data (credit reports, official proof of income, salary history and others) they require to make informed lending decisions. As a result, SMEs don’t get access to funding and have to rely on informal lenders who are notorious for charging exorbitant interest rates. According to a Boston Consulting Group and Omidyar Network report, around 40 percent of India’s MSME lending is done through the informal sector. The need of the hour, therefore, is the incorporation of tech-driven tools to identify genuine borrowers, underwrite risk and monitor it close to real-time, thereby eliminating biases in the credit decision-making process, enabling cost savings and reducing the loan processing time significantly.
AI-based Credit Underwriting for Faster Loan Processing
Modern technologies can automate and streamline the credit underwriting process. Financial institutes have traditionally been using a manual process for scoring and approving or rejecting loans, wherein analysts score each loan against a set of financial metrics that are in place to determine the acceptability of the borrower. The manual process of credit scoring is time-consuming and often leads to mistakes and biased decisions. In contrast, an AI-powered credit underwriting model combines traditional data with alternative data, which includes the borrower’s digital footprint, daily transactions, payments history and even legal case history, to provide accurate credit assessment in near realtime. Besides facilitating faster processing and approval of loans, AI-based scoring systems enable credit underwriters to focus on more complex issues that might requirehuman intervention.
Besides facilitating faster processing and approval of loans, AI-based scoring systems enable credit underwriters to focus on more complex issues that might require human intervention
Integration of Machine Learning for Accurate Credit Risk Analysis
Credit-risk analysis allows financial institutes to assess the possibility of the borrower’s failure to repay the loans and the amount of loss caused by the same. If done manually, credit-risk analysis can not only take up to days, but also is more prone to mistakes. This is where machine learning has a crucial role to play. ML-based credit analysis models can identify complex, nonlinear patterns within large sets of data, thereby enabling more accurate credit-risk analysis.Such analysis can help lenders to understand the true credit-worthiness of the borrower and reduce default cases to a great extent. Some of the powerful algorithms that banks, Fintechs and NFBCs can use to weed out bad cases early are artificial neural networks, random forest and boosting.
How does this benefit the SMEs?
Lending to small and medium enterprises poses a major challenge for lenders in India. That’s because lenders have little or no access to data (credit reports, official proof of income, salary history and others) they require to make informed lending decisions. As a result, SMEs don’t get access to funding and have to rely on informal lenders who are notorious for charging exorbitant interest rates. According to a Boston Consulting Group and Omidyar Network report, around 40 percent of India’s MSME lending is done through the informal sector. The need of the hour, therefore, is the incorporation of tech-driven tools to identify genuine borrowers, underwrite risk and monitor it close to real-time, thereby eliminating biases in the credit decision-making process, enabling cost savings and reducing the loan processing time significantly.